Cebu’s Data Challenges and Policy-Making: Policy-making Needs Improved Data, Cebu Guv Says
Cebu, a thriving province in the Philippines, faces significant challenges in leveraging data for effective policy-making. Governor Gwendolyn Garcia’s recent statement highlighting the need for improved data underscores the urgency of addressing existing data gaps and strengthening data management systems. This article explores Cebu’s current data landscape, the impact of data deficiencies on policy outcomes, strategies for improving data collection and management, data analysis and utilization, and the importance of collaboration and capacity building.
Cebu’s Current Data Landscape, Policy-making needs improved data, Cebu guv says
Data collection and management in Cebu’s policy-making processes are currently fragmented and lack standardization. Key data gaps exist across various sectors, hindering the development and implementation of effective policies. Compared to other regions in the Philippines, such as Metro Manila, or internationally, Cebu lags in terms of data availability, accessibility, and utilization for evidence-based policy-making. The existing data infrastructure is insufficient, with limited interoperability between different government agencies and a lack of robust data storage and management systems.
Data Type | Source | Reliability | Use in Policy |
---|---|---|---|
Demographic Data (Population, Age, Gender) | Philippine Statistics Authority (PSA), Local Civil Registries | Generally Reliable, but may have inconsistencies across sources | Population planning, resource allocation, social welfare programs |
Economic Data (GDP, Income Levels, Poverty Rates) | National Economic and Development Authority (NEDA), Local Government Units (LGUs) | Moderate Reliability, subject to revision and potential underreporting | Economic development planning, investment decisions, poverty reduction programs |
Social Data (Education Levels, Health Indicators, Crime Rates) | Department of Education (DepEd), Department of Health (DOH), Philippine National Police (PNP) | Varied Reliability depending on data collection methods and reporting accuracy | Social welfare programs, education planning, public safety initiatives |
Environmental Data (Waste Generation, Air Quality, Water Resources) | Environmental Management Bureau (EMB), LGUs | Improving, but often lacks comprehensive coverage and real-time monitoring | Environmental protection policies, disaster risk reduction, sustainable development planning |
Impact of Data Deficiency on Policy Outcomes
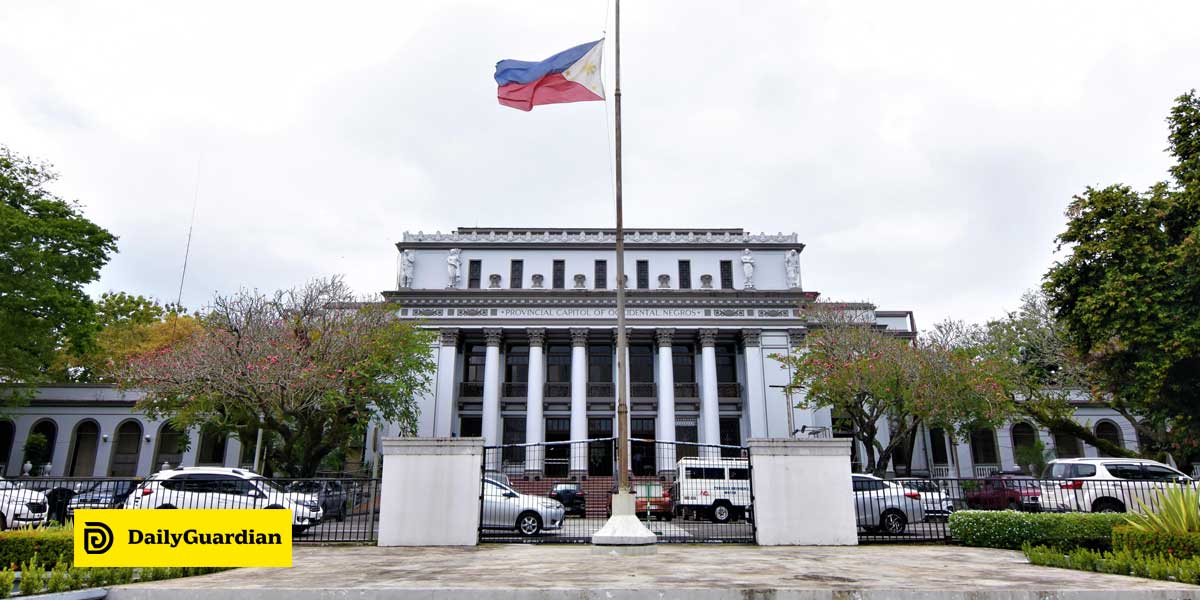
Inadequate data significantly impacts the effectiveness of policies in Cebu. For example, poor-quality data on poverty rates can lead to inefficient allocation of resources for social welfare programs. Similarly, incomplete data on traffic patterns can result in ineffective transportation planning. The consequences include financial losses due to wasted resources, social inequities due to misdirected programs, and environmental damage due to poorly informed decisions.
- Health: Inaccurate health data can lead to insufficient resource allocation for disease prevention and control, resulting in higher morbidity and mortality rates.
- Education: Lack of comprehensive data on student performance can hinder the development of effective educational strategies, leading to lower educational attainment.
- Infrastructure: Inadequate data on infrastructure needs can result in poorly planned projects, leading to wasted resources and ineffective infrastructure development.
- Economic Development: Insufficient economic data can lead to flawed economic policies, hindering economic growth and job creation.
Improving Data Collection and Management
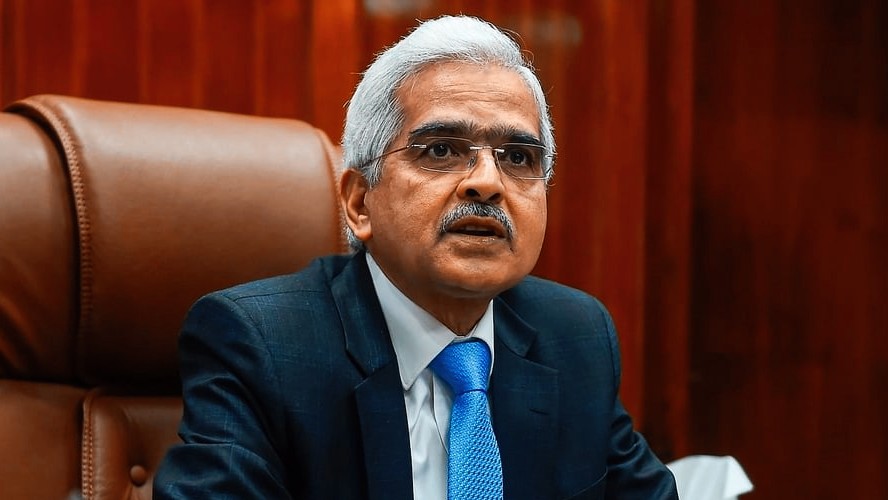
A comprehensive strategy for enhancing data collection in Cebu should involve standardizing data collection methods across government agencies, adopting modern technologies for data capture and management, and implementing robust data security and privacy protocols. This will require investment in new data infrastructure, training for personnel, and the establishment of clear data governance structures. Potential funding sources include national government allocations, international development assistance, and private sector partnerships. Data governance will ensure data quality, accessibility, and responsible use.
Data Analysis and Utilization for Policy
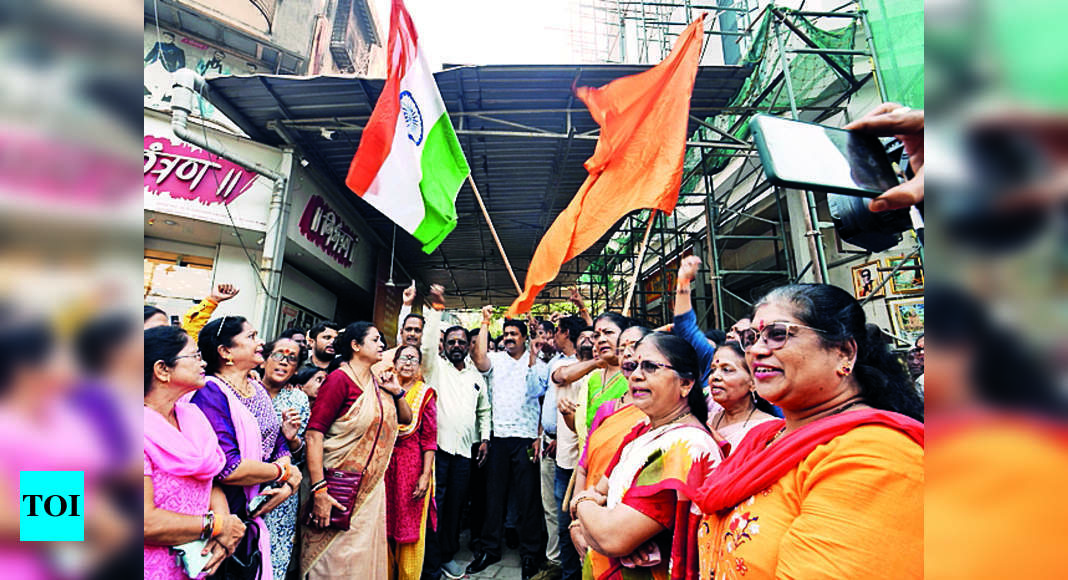
Evidence-based policy-making in Cebu requires the use of appropriate data analysis methods. Statistical modeling can be used to analyze trends and patterns in data, while predictive analytics can be used to forecast future outcomes. Clear and effective communication of data-driven insights is crucial for influencing policy decisions. Data visualization tools, such as interactive dashboards, can effectively communicate complex information to policymakers and the public.
A hypothetical data visualization showing the correlation between poverty rates and access to healthcare could utilize a scatter plot. The X-axis would represent poverty rates (percentage), and the Y-axis would represent access to healthcare (percentage of population with access). Each point on the plot would represent a municipality or barangay in Cebu, with color-coding indicating the level of healthcare access. This visualization would clearly illustrate the relationship between poverty and healthcare access, enabling policymakers to target interventions effectively.
Collaboration and Capacity Building
Effective data-driven policy-making in Cebu requires strong collaboration among various stakeholders, including government agencies, civil society organizations, and the private sector. A collaborative framework should be established for data sharing, expertise exchange, and joint project implementation. Training programs should be developed to enhance the capacity of policymakers and staff to effectively utilize data in their work. This will foster a culture of data-driven decision-making within Cebu’s government.
Stakeholder | Role | Data Needs | Contribution to Policy |
---|---|---|---|
Provincial Government of Cebu | Overall coordination, policy development, resource allocation | Comprehensive data across all sectors | Policy formulation, implementation, monitoring, and evaluation |
LGUs (Municipalities and Cities) | Data collection at local level, implementation of programs | Local-level data, relevant to specific needs | Implementation of policies, feedback on policy effectiveness |
PSA | Data collection and dissemination, statistical analysis | Demographic, economic, and social data | Provision of reliable data for policy development |
Civil Society Organizations (CSOs) | Community engagement, data collection, advocacy | Data related to specific social issues | Community insights, monitoring and evaluation of programs |
Private Sector | Data provision, technical expertise, funding | Data related to business activities, investments | Economic data, technical expertise, funding for data infrastructure |